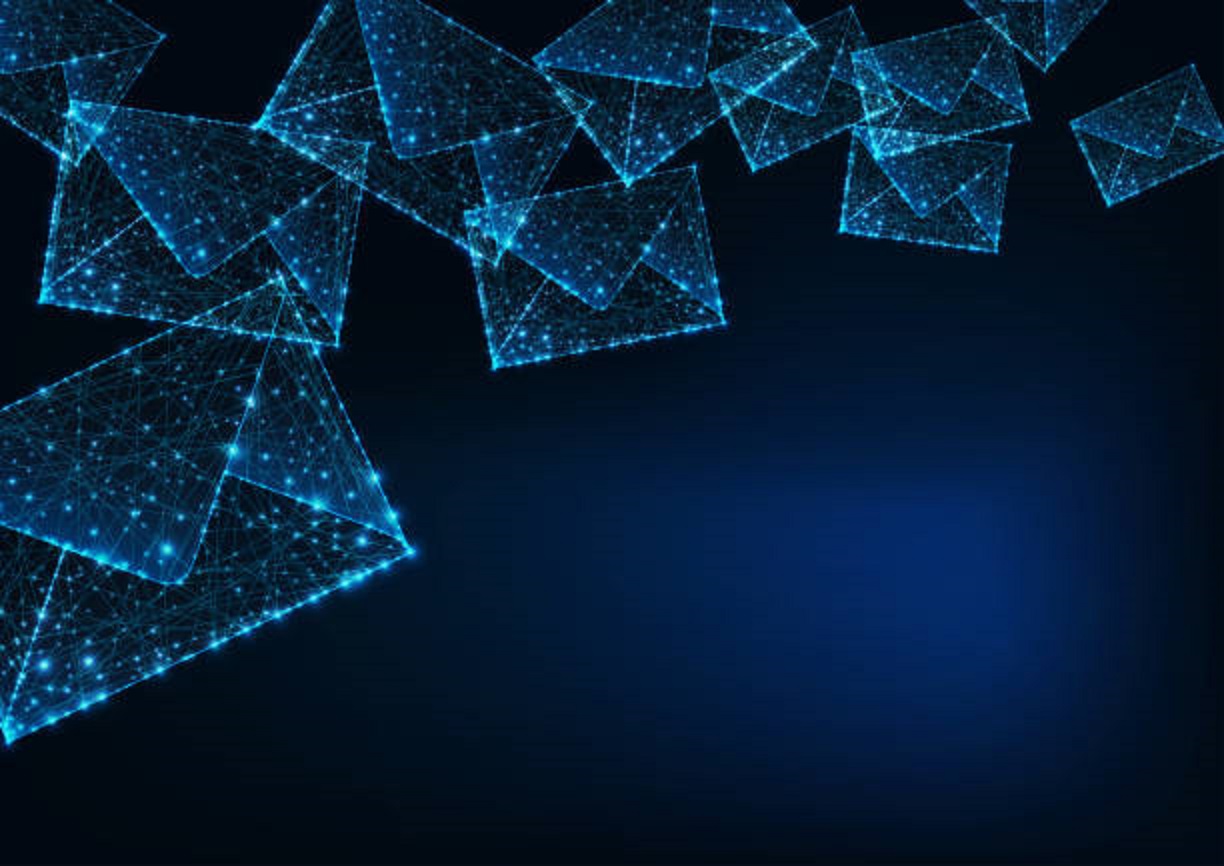
An NLP EHR integration, known as eCOV, accurately identified acute COVID-19 cases with 94% sensitivity from patient portal messages, according to a study. Prompt responses to patient messages led to increased antiviral prescriptions within the recommended treatment window. The integration of NLP models in EHR systems can help triage urgent messages and improve reporting accuracy. It also enables timely remote care for COVID-19 patients, reducing hospitalization rates and burden on healthcare systems, though further analysis is needed to assess the true clinical impact.
According to a study published in JAMA Network Open, the integration of natural language processing (NLP) models into electronic health records (EHR) has proven effective in quickly identifying and accurately reporting COVID-19 cases.
The study focused on utilizing an NLP model called eCOV to triage self-reported positive COVID-19 cases in real-time through the patient portal. The model demonstrated a sensitivity of 94% in identifying acute cases of COVID-19 from patient messages.
The research also revealed that when healthcare providers responded to patient messages promptly, patients were more likely to receive timely antiviral prescriptions within the recommended five-day treatment window.
The authors emphasized the significance of health IT tools like NLP models in efficiently triaging urgent patient messages, particularly in large healthcare systems that handle a high volume of patient portal messages daily.
The researchers noted that the timing of physician response to patient messages could determine the appropriate administration of oral antiviral treatment, potentially leading to reduced hospitalization and mortality rates.
Additionally, the NLP model facilitated timely care by enabling patients with positive COVID-19 test results to report them through the EHR messaging system. This allowed primary care physicians (PCPs) to remotely treat some COVID-19 patients, reducing hospitalization rates, minimizing infection risk for healthcare workers, and relieving pressure on the healthcare system.
Furthermore, the NLP model significantly improved the accuracy of case reporting, as only 2.2% of the identified COVID-19 cases were previously documented in the structured EHR data elements.
The authors highlighted that by accurately classifying patient messages and improving access to treatment, NLP integration into EHR systems has the potential to enhance clinical outcomes while alleviating the burden on the healthcare system. However, further analysis is necessary to quantify the true clinical impact of this integration.
The study’s limitations included the absence of visual validation of test results and the inability to verify treatment adherence or prescriptions from external facilities. Nevertheless, the researchers suggested that the underreporting of cases likely outweighed false-positive reporting.
Additionally, the researchers acknowledged that symptom onset could not be systematically evaluated, meaning that some patients identified as potential candidates for antiviral treatment might have surpassed the treatment window by the time their message was sent.