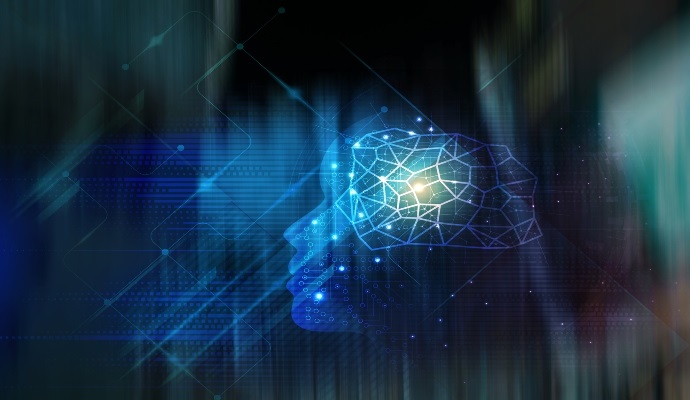
Pioneering Research – Wearable Biosensors Anticipate Autism-Related Aggression. Northeastern University’s study showcases the potential of machine learning coupled with biosensor data in forecasting aggressive behavior among autistic inpatients. This groundbreaking approach extends prior successes, enabling a three-minute pre-warning of aggressive episodes. The research, encompassing 86 participants with confirmed autism diagnoses, demonstrates the viability of logistic regression as the foremost predictor, achieving an impressive 0.80 area under the receiver operating characteristic curve. The findings propose innovative just-in-time adaptive interventions, promising a transformative impact on the understudied and underserved autism population. These advancements align with emerging technologies supporting ASD diagnosis, reinforcing the potential for personalized, tech-driven approaches in mental health care.
Advances in autism care have burgeoned with the fusion of wearable technology and predictive analytics. Northeastern University’s recent study underscores this transformative shift, illuminating the convergence of wearable biosensors and machine learning to forecast aggression among inpatient youths with autism spectrum disorder (ASD). Building upon prior successful trials, this research aims to extend the prediction window, heralding a substantial three-minute lead time in anticipating aggressive behavior. The study’s comprehensive analysis involving 86 diagnosed participants underscores logistic regression as a potent tool, achieving impressive predictive accuracy. These findings usher in a new era of potential interventions, poised to revolutionize support strategies for the often marginalized autism population.
The integration of wearable technology with advanced predictive analytics is revolutionizing the field of mental health care, particularly in assessing and preempting aggressive behavior among inpatients with autism spectrum disorder (ASD). Recent research conducted by Northeastern University demonstrates the potential of a wearable biosensor coupled with machine learning algorithms to anticipate instances of aggression in youths with autism before they occur, marking a significant stride in improving care for this demographic.
In the study published in JAMA Network Open, researchers investigated the efficacy of a wearable biosensor in gathering physiological data from inpatient youths diagnosed with ASD. The device monitored various physiological parameters like cardiovascular activity, electrodermal activity, and motion, which were subsequently analyzed through machine learning techniques. The primary objective was to ascertain whether these collected data sets could predict instances of aggressive behavior in advance, thus allowing for timely intervention and support.
The research was built upon earlier work by the same university team that successfully predicted aggressive behavior toward others a minute before it occurred, based on three minutes of recorded peripheral physiological and motion signals from 20 youths with autism. This newer study aimed to extend this prediction timeframe further back.
The study enrolled 86 participants from primary care psychiatric inpatient hospitals, all diagnosed with autism and exhibiting self-injurious behavior, emotion dysregulation, or aggression toward others. The participants wore a commercially available biosensor while researchers conducted real-time coding of aggressive behavior. The recorded data underwent rigorous analysis, involving feature extraction, data preprocessing, and various machine learning algorithms such as logistic regression, support vector machines, neural networks, and domain adaptation.
Of the 86 participants, 70 were included in the final analysis due to certain limitations faced by the remaining individuals in wearing the biosensor or early discharge. The observational coding sessions accumulated to a substantial 497 hours, during which researchers recorded thousands of instances of aggressive behavior, including self-injurious behavior, emotion dysregulation, and aggression toward others.
The results showcased the efficacy of logistic regression as the most reliable classifier, exhibiting the ability to predict aggressive behavior three minutes in advance with a mean area under the receiver operating characteristic curve of 0.80. This groundbreaking finding suggests the potential of biosensor data coupled with machine learning in addressing the challenges faced by a significant segment of the autism population that is often overlooked and underserved.
The implications of this study extend beyond its immediate findings, opening avenues for the development of just-in-time adaptive intervention mobile health systems. Such systems could revolutionize the way preemptive interventions are implemented, offering timely support to individuals prone to aggressive behavior due to their autism diagnosis.
This innovative approach utilizing wearable biosensors and machine learning to predict aggressive behavior in advance aligns with other advancements in supporting individuals with ASD. For instance, researchers in Atlanta developed an eye-tracking diagnostic tool based on biomarkers, aiming to diagnose ASD by monitoring children’s gaze during social interaction videos. Additionally, a University of California, Davis researcher received NIH funding to explore the efficacy of telehealth in assessing ASD among infants, potentially enabling earlier diagnosis and intervention.
The synergy between wearable biosensors and predictive analytics marks a watershed moment in autism care. Northeastern University’s study heralds a paradigm shift by harnessing machine learning to anticipate aggressive behavior among ASD-diagnosed inpatients. The study’s remarkable success in achieving a three-minute prediction horizon unveils a promising trajectory for timely interventions. The envisioned just-in-time adaptive health systems promise personalized support, addressing the unmet needs of the autism community. Complemented by parallel innovations in ASD diagnosis through technological tools, these advancements foreshadow a future of tailored, technology-centric mental health care approaches. This amalgamation of wearable tech and predictive analytics represents a transformative stride toward enhanced well-being and support for individuals navigating autism spectrum disorders.