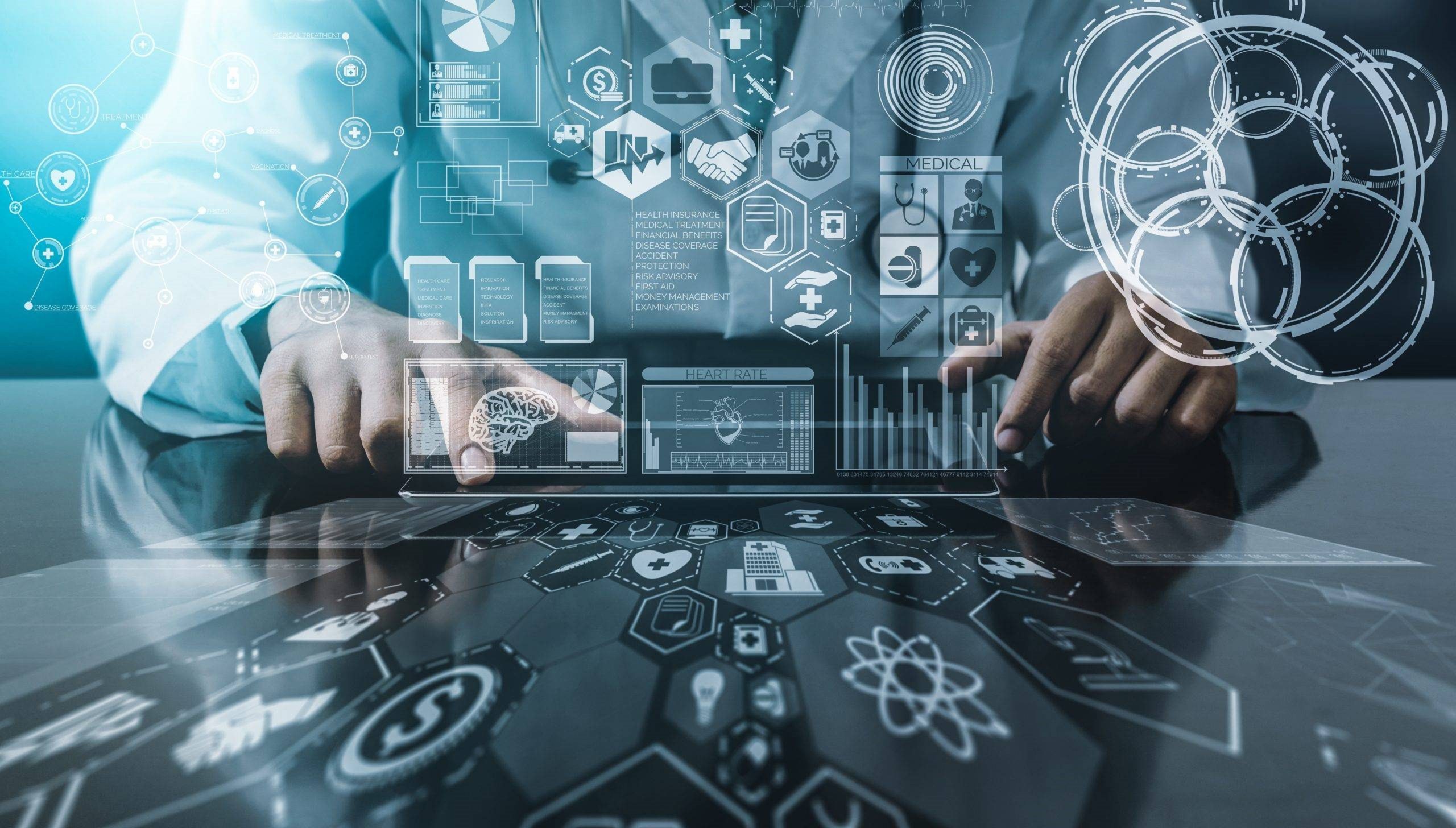
In healthcare analytics, data storage, management, and analysis are crucial stages. Data storage involves preserving digital information using file, block, or object storage, while management ensures data quality and interoperability. Analytics comprises descriptive, diagnostic, predictive, and prescriptive analysis. These processes are critical for improving healthcare services and patient outcomes. Choosing the right storage solution, developing a data management plan, and harnessing advanced technologies like machine learning and natural language processing enhance healthcare data utilization. Subsequent steps include data visualization, interpretation, and disposal, completing the healthcare data lifecycle.
In the realm of healthcare analytics, data storage, management, and analysis are critical stages. But what exactly do these stages entail?
The healthcare data lifecycle guides analytics projects across various industries, and healthcare is no exception. Healthcare stakeholders must comprehend each phase of this cycle, including data generation, collection, processing, storage, management, analysis, visualization, interpretation, and disposal, to ensure the success of their analytics endeavors.
This article is part of a series dedicated to exploring the healthcare data lifecycle. In a previous installment, we delved into data generation, collection, and processing. Now, we’ll focus on data storage, management, and analysis.
Data Storage
Data storage encompasses the use of magnetic, optical, or mechanical media to record and preserve digital information for ongoing or future operations, as defined by IBM. This process involves computers (terminals) connecting to storage devices directly or through a network. Users can then instruct terminals to access and store data within these storage devices.
There are three primary forms of data storage: file, block, and object.
1. File storage is the most familiar form, used in both personal and professional settings. It utilizes a hierarchical method to organize data into files, folders, directories, and subdirectories.
2. Block storage stores data in separate blocks, each with a unique identifier, prioritizing efficient data transfer.
3. Object storage is designed for unstructured data, such as sensor data, emails, photos, videos, and more.
Data storage devices fall into two main types: direct area storage (DAS) and network-based storage.
– DAS refers to storage directly connected to or near the accessing terminal, including flash drives, solid-state drives (SSDs), and hard disk drives (HDDs).
– Network-based storage enables multiple terminals to access stored data through a network, providing advantages like off-site storage, improved data protection, and data sharing.
Health data storage options typically include on-premise, cloud, or hybrid solutions. The adoption of cloud technologies in healthcare has been on the rise, with healthcare organizations considering public, private, or hybrid cloud storage based on their specific needs, infrastructure, and scalability requirements.
While data storage tools offer numerous benefits, they also pose challenges related to privacy, security, scalability, and cost, along with occasional outages. Solutions like object storage and blockchain are being explored to address these limitations.
Data Management
Data management involves validating, organizing, protecting, maintaining, and processing data to ensure accessibility, reliability, and quality. In healthcare, proper data management is crucial for research integrity and improved patient outcomes.
Health systems can employ data management systems to clean, standardize, and unify data, enhancing interoperability and internal analytics projects.
Developing a data management plan is essential at this stage. It outlines data types, storage, security, privacy, and sharing considerations. Stakeholders must also address weaknesses in their data management systems to improve research and clinical care.
Data operating and storage solutions lay the foundation for an effective healthcare data management system. Integration with a vendor-neutral archive (VNA) is recommended to ensure compatibility with various data management solutions.
Data management has already proven valuable in addressing healthcare challenges, such as opioid crisis research, where data management and stewardship expertise play a crucial role.
Analysis
Data analytics, defined by AHIMA, involves using quantitative and qualitative techniques to explore data for trends and patterns. It is closely related to informatics, which focuses on applying information gathered from data analysis to improve healthcare services and patient outcomes.
Healthcare data analytics encompasses four categories: descriptive, diagnostic, predictive, and prescriptive analytics.
1. Descriptive analytics answers the question “What happened?” by identifying and describing trends in data.
2. Diagnostic analytics delves into why certain trends or anomalies identified in descriptive analytics occurred.
3. Predictive analytics forecasts future patterns based on historical data, requiring significant resources and data.
4. Prescriptive analytics guides improving performance based on insights from the previous analytics types, requiring substantial data and computational capabilities.
Various technologies, including machine learning, deep learning, cognitive computing, natural language processing, and semantic computing, support these analytics types, addressing challenges in healthcare, from population health management to value-based care.
Following the analysis stage, stakeholders can progress to data visualization, interpretation, and disposal, which we will explore in the final part of this series.