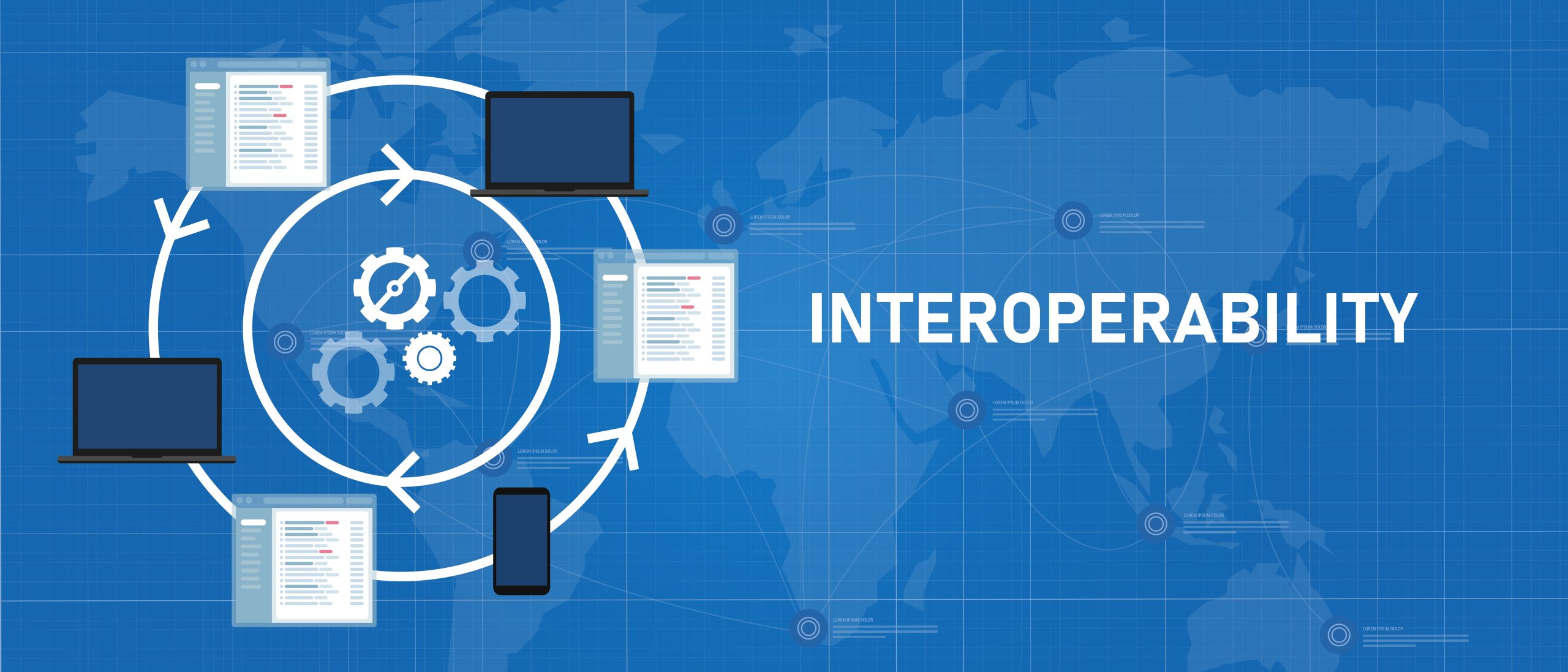
Standardized EHR data quality guidelines are crucial for enhancing interoperability in healthcare. Current research lacks consistent assessment methods, hindering efficiency. Studies highlight the need for automation and flexible tools to evaluate dimensions like completeness, correctness, concordance, plausibility, currency, conformance, and bias. Biased EHR data can negatively impact patient care and machine learning models. Common data models (CDMs) support interoperability and reusable DQA tools. Implementing these guidelines will ensure high-quality EHR data and promote seamless data exchange across applications.
Ensuring high-quality electronic health record (EHR) data is essential for achieving interoperability within the healthcare industry. However, the lack of standardized guidelines and tools for assessing EHR data quality has been a significant barrier to this goal, as highlighted in a study published in JAMIA.
Enhancing Efficiency and Interoperability:
The study emphasizes the need for EHR data quality assessment (DQA) guidelines to improve the efficiency, transparency, comparability, and interoperability of data quality assessment. To evaluate changes in DQA methodologies, researchers expanded on a 2013 literature review of EHR data quality assessment approaches and tools. The original review identified five dimensions of EHR data quality:
1. Completeness: Ensuring the presence of comprehensive data in the EHR.
2. Correctness: Verifying the accuracy and truthfulness of data in the EHR.
3. Concordance: Assessing the consistency between elements within the EHR and other data sources.
4. Plausibility: Evaluating the coherence of EHR data within the broader medical context.
5. Currency: Determining the accuracy and timeliness of recorded EHR data.
Findings and Recent Developments:
By reviewing 103 papers published between 2013 and April 2023, the researchers observed an increase in the number of dimensions assessed per paper compared to 2013. The most commonly assessed dimension was completeness, followed by correctness, concordance, plausibility, and currency.
The study also introduced two additional dimensions to the data quality assessment framework:
1. Conformance: Examining compliance with a predefined representational structure.
2. Bias: Identifying instances of non-random missingness in data, potentially leading to biased samples.
The Impact of Biased EHR Data:
The presence of bias in EHR data can have adverse effects on patient care, particularly when machine learning models are employed in clinical decision support tools. For instance, biases related to data completeness among sicker patients can lead to skewed results and potentially compromise patient outcomes.
Towards Standardization and Automation:
The study highlights the need for consistent and efficient DQA methods rather than relying on project-specific approaches. Automation of DQA processes emerges as a crucial theme, mentioned in various opinion pieces and tools, given the widespread use of EHR data for downstream analysis. The authors emphasize the importance of striking a balance between scalability and task-specificity when considering tools for assessing data quality.
The Role of Common Data Models (CDMs):
In addition to automation, the authors suggest leveraging common data models (CDMs) to support interoperability and the development of reusable DQA tools. As data requirements may vary across different systems and projects, adopting flexible tools becomes crucial for assessing data quality effectively.
The establishment of EHR data quality guidelines is paramount to drive interoperability in the healthcare industry. By addressing the dimensions of data quality, incorporating conformance and bias assessments, and embracing automation and CDMs, stakeholders can promote the consistent and standardized evaluation of EHR data quality across diverse applications.