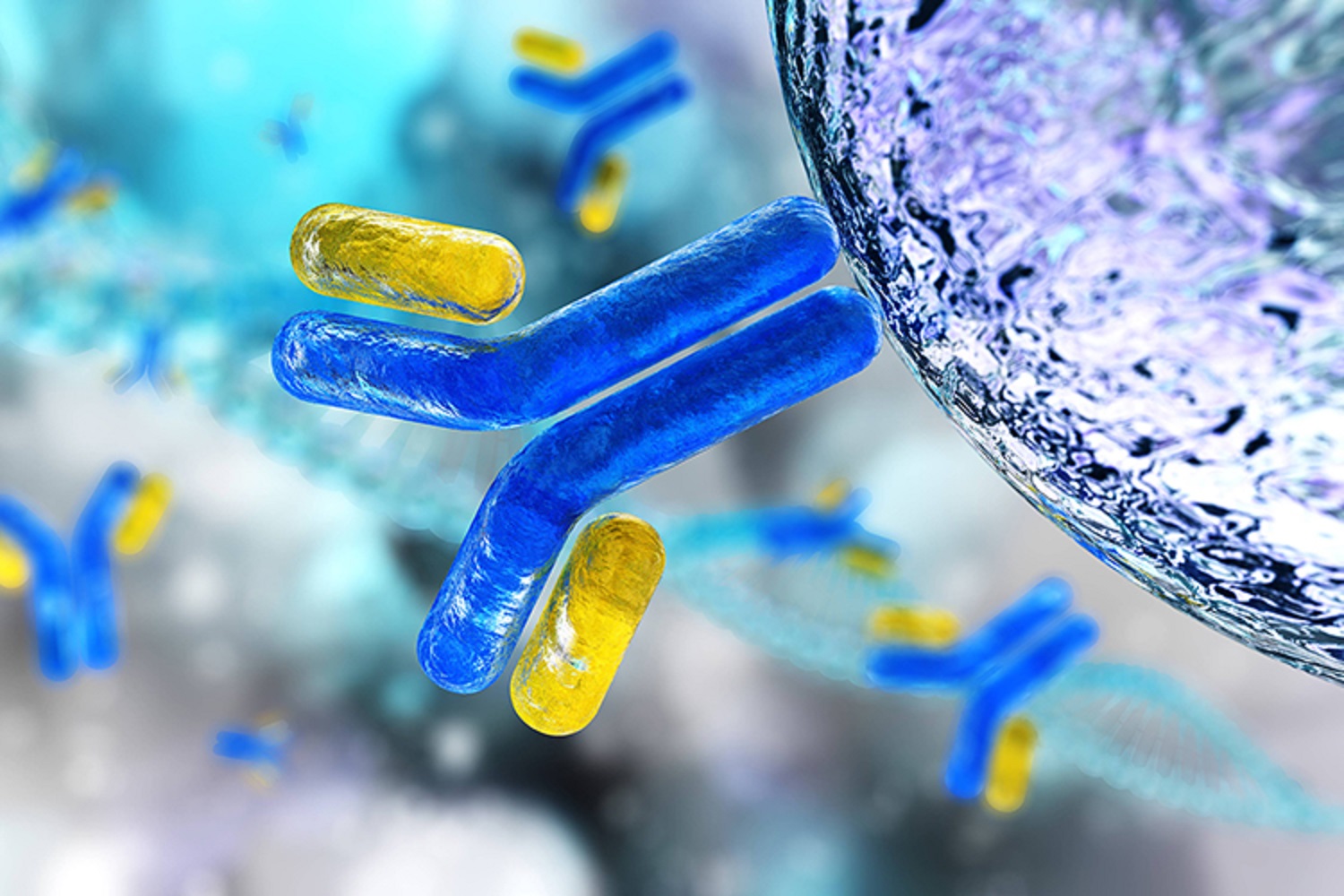
Researchers at the University of Michigan have utilized machine learning to optimize therapeutic antibodies. These antibodies are crucial for fighting diseases but often bind to unintended molecules, reducing their effectiveness. The ML model identifies problem areas within antibodies and suggests corrections, significantly expediting the optimization process. This breakthrough may revolutionize drug development, with biotech companies already integrating ML into antibody optimization efforts. Additionally, Ohio State University researchers have introduced an AI framework called G2Retro to streamline chemical reaction generation in drug development.
A recent study published in Nature Biomedical Engineering reveals a breakthrough in therapeutic antibody optimization by researchers at the University of Michigan. They have harnessed the power of machine learning (ML) to enhance the effectiveness of these antibodies and reduce their tendency to bind with non-target molecules.
Therapeutic antibodies play a crucial role in drug development, activating the immune system to combat various diseases. However, a significant challenge has been their tendency to bind with molecules unrelated to the targeted disease or even with other antibodies, compromising their efficacy.
The ML tool developed by the researchers aims to tackle this issue by identifying problematic regions within antibodies and suggesting potential corrections.
Lead author Peter Tessier, Ph.D., the Albert M. Mattocks Professor of Pharmaceutical Sciences, Chemical Engineering, and Biomedical Engineering at the University of Michigan, explains, “We can utilize these models to pinpoint trouble spots within antibodies and make precise adjustments to correct the issues without introducing new ones. These models are versatile, applicable to existing antibodies, antibodies in development, and even those yet to be created.”
Antibodies operate by binding to antigens—markers on the surface of various entities such as bacteria, viruses, proteins, allergens, parasites, tumor cells, and normal cells, aiding in identifying foreign agents within the body.
Once an antibody binds to an antigen, it can trigger the body’s immune cells to attack the foreign invader or directly neutralize viruses or cells.
Therapeutic antibodies, meticulously developed and optimized in laboratories, are engineered to combat severe and challenging conditions like Alzheimer’s, Parkinson’s, and colorectal cancer by targeting specific antigens.
However, these antibodies often engage in unwanted interactions with each other and non-antigen molecules once administered, diminishing their effectiveness.
Tessier emphasizes, “The ideal antibody should simultaneously achieve three objectives: bind tightly to its intended target, repel other antibodies, and disregard unrelated substances in the body.”
Antibodies failing to meet these criteria are unlikely to become successful drugs, highlighting the necessity for improved antibody optimization.
To bridge this gap, the researchers scrutinized 80 clinical-stage molecules, revealing that 75 percent exhibited undesired interactions with the wrong molecules or other antibodies, or both.
Modifying an antibody’s amino acids and three-dimensional structure could potentially address these issues, but each antibody possesses numerous alterable amino acid positions, and these adjustments might introduce new problems during laboratory testing.
Emily Makowski, the study’s first author and a recent Ph.D. graduate in pharmaceutical sciences at the University of Michigan, states, “Exploring all the changes for a single antibody takes about two workdays with our models, significantly shorter than the months required for experimental measurements of each modified antibody.”
In response to these challenges, the research team turned to machine learning. They trained algorithms using experimental data from the 80 clinical-stage antibodies to identify modifications in each antibody that could align with Tessier’s three criteria.
Overall, the ML model demonstrated an impressive accuracy rate of 78 to 88 percent in identifying potential modifications, which could significantly reduce the number of necessary antibody changes during laboratory optimization.
The researchers anticipate that machine learning will play a pivotal role in the future of drug development. Biotechnology companies are already leveraging these tools to optimize therapeutic antibodies and advance drug development efforts.
Tessier remarks, “Some companies have developed antibodies with promising biological activity but anticipate challenges when using them as drugs. That’s where we come in, pinpointing specific areas in their antibodies that require adjustments. We are already assisting several companies in this endeavor.”
Other institutions are also exploring how artificial intelligence (AI) can drive innovation in drug development. In a recent development, researchers at Ohio State University introduced a generative AI framework called G2Retro. This tool streamlines retrosynthetic analysis, a critical step in drug development, by using ML to generate reactant structures for synthesizing target molecules efficiently.